
How to Predict B2B SaaS Market Evolution Before Your Competitors (Part 2)
Today’s agile product methods optimise for iteration, not foresight. Deductive Innovation shows how to identify and develop the optimal B2B SaaS solution – before your market converges there.
👋 Hi, it’s Antti LK and this is the second article in a 4-part series on predicting market evolution in B2B SaaS. Read Part 1 here. Subscribe now to keep following the series!
Agile product development methodologies are iterative approaches to help you efficiently climb whatever hill you are on. But what if you are on the wrong mountain entirely? This article explores how to identify the highest peak in your market landscape before you start climbing – and why today’s best practices won’t get you there.
In the previous article of this series, we explored how markets tend to converge toward optimal solutions that address the complete set of customer problems with minimal complexity. This concept of “optimality” is crucial: it doesn’t mean having the most features or the sleekest UI, but rather solving the whole underlying customer problem as simply and directly as possible. The solutions that ultimately dominate markets are those that maximise value while minimising unnecessary complexity.
We also examined five mental barriers that prevent people from seeking optimal solutions:
The Relativism Trap (believing there are no best solutions)
The Measurement Challenge (the difficulty of evaluating solutions without building them)
The Kilimanjaro Effect (when mediocre solutions appear equally good)
The Cost Fallacy (assuming superior solutions cost more to build)
The Impermanence Fallacy (the myth that customer needs change rapidly)
These barriers keep most companies trapped in cycles of iterative improvement rather than looking for transformative opportunities. In this article, we’ll examine why current methodologies struggle with market prediction and introduce a systematic approach that helps you overcome these barriers and see where your market is heading years in advance.
The Limitations of Current Approaches
The product development approaches popular today, like agile, lean startup, and design thinking, all embrace iteration as a core principle. Agile’s “responding to change over following a plan”1 makes sense when you don’t have confidence in what the best plan would be.
While these approaches excel at incremental improvement, they have a critical gap: they provide very little guidance on how to identify the best possible solution – the global optimum in the solution space. Their fundamental limitations include:
They are primarily responsive, adjusting based on feedback rather than anticipating optimal destinations
They can lead to local maximums rather than finding global ones
They often consume significant resources through repeated iterative cycles
They offer limited predictive capability for market evolution
They struggle particularly with complex B2B software where the solution space is vast, and testing and iterating the entire product is prohibitively expensive
As Peter Thiel observes in his influential book Zero to One:2
Making small changes to things that already exist might lead you to a local maximum, but it won’t help you find the global maximum.
He then points to the need for more visionary thinking in building new products and businesses:
Iteration without a bold plan won’t take you from 0 to 1.
But how do we develop these “bold plans” systematically? Is the only option to rely on a rare creative genius with visionary insights from mysterious origins (think Steve Jobs)?
Why Finding the Best Solution is So Challenging
Finding the best solution to complex customer problems is extraordinarily difficult. That is the reason today’s best practices rely heavily on iteration, experimentation, and feedback cycles.
I like to compare this to the evolution of artillery. In medieval times, gunners made their best guess at aiming a cannon, fired, observed the results, and adjusted. This trial and error approach wasn’t because they were less intelligent than modern artillerymen. It was necessary because they lacked the necessary theoretical knowledge to calculate trajectories correctly.3 Without a robust explanatory theory of ballistics, iteration was the “best practice.”
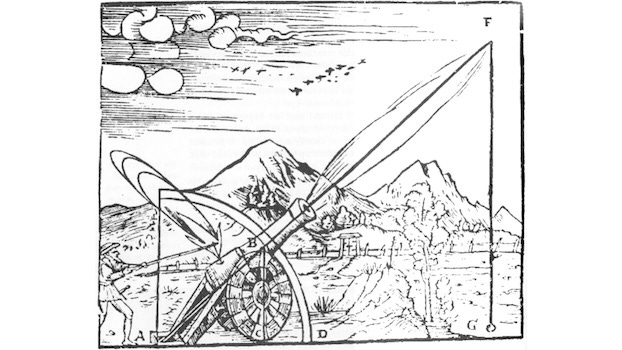
Today’s artillery can hit distant targets with remarkable precision, often without any iteration, because we developed the physics of ballistics, differential equations, and computational methods to model projectile motion. The critical advancement wasn’t better cannons; it was better explanations.
Product development for complex B2B software is still largely in its “medieval” stage. We lack widely understood, predictive theories that could explain the relationship between customer problems, solutions, complexity, and value creation. Just as artillerymen once relied on trial and error, today’s product teams rely on iteration because they lack explanatory theories about optimal solutions. In the absence of such explanatory theories, thoughtful iteration is a reasonable response. But it shouldn’t be the end state of our field.
This evolution from trial and error to explanatory theories is a pattern of human progress across disciplines. Advancements come not from iteration, but from creatively developing better explanations that can predict outcomes in a large variety of situations, and then exposing those explanations to criticisms and testing.
Just like Newton’s discovery of the laws of motion and differential equations led to more accurate artillery, his theory of optics led to inventing a better telescope using mirrors instead of lenses. Maxwell’s theory of electromagnetism explained electricity and magnetism and predicted the existence of electromagnetic waves, which led to the invention of radio. Discovery of quantum mechanics led to the invention of the transistor.
When it comes to product development, we need to be able to explain the causal relationships between customer problems, aspects of software solutions, and customer value. This understanding will enable any product team to systematically identify optimal solutions, not just those blessed with extraordinary intuition or luck. This is where Deductive Innovation comes in.
Deductive Innovation: Finding the Global Maximum Systematically
The Core Concept: From Iteration to Prediction
Deductive Innovation represents a fundamental shift in approaching product development: moving from iteration toward prediction, from guesswork toward knowledge.
The central promise is: What if we could consistently identify optimal solutions before our competitors? Not just solutions that are marginally better, but those that are transformatively better – the ones that will eventually dominate the market?
As Peter Thiel observes in Zero to One:
As a good rule of thumb, [a solution] must be at least 10 times better than its closest substitute in some important dimension to lead to a real monopolistic advantage. Once you’re 10x better, you escape competition.
This kind of 10x improvements are achievable in the complex world of B2B software where current solutions are often far from optimal. But achieving this level of improvement requires a fundamentally different approach to product development. Thiel had a suggestion:
You can make a 10x improvement through superior integrated design.
Deductive Innovation is a structured approach to creating exceptional integrated designs specifically in the complex B2B software space. I have developed it over 20+ years of working with software products across multiple industries. It solves the shortcomings of common product development and design methods when it comes to complex software products that support customers’ functional needs.
The Four-Step Deductive Innovation Process
Breaking from the inherent limitations of iterative approaches, Deductive Innovation follows a systematic sequence to designing an overall solution concept before resources are spent on building it.
Rather than immediately diving into customer problem discovery, which itself requires resources, we first determine if an opportunity merits that investment:
Measure improvement potential before investing in problem discovery (Deductive Evaluation): We first evaluate if a product opportunity has sufficient potential to create customer value compared to existing solutions. This analytical measurement approach helps us focus only on opportunities with potential for at least 5x-10x improvements in Problem-Solution Fit.
Discover customer problems with sufficient depth for deduction (Deductive Discovery): For opportunities with high potential, we systematically discover, map, understand and validate the customer problem space in a very particular way. The key criterion is gathering sufficient facts about the customers’ world to make the next stage possible.
Design the solution by deduction from customer problems (Deductive Design): Using this detailed problem understanding, we systematically deduce the optimal solution, potentially years before competitors.
Build the solution incrementally, starting where most customer value can be created with least resources, taking into account the dependencies between different parts of the overall optimal solution, and prototyping technologies to reduce feasibility risk.
Deductive Innovation reverses the traditional approach. Instead of ideating and building a solution (or even a prototype) and iterating based on feedback, it first measures value creation potential, then builds the specific knowledge about customer problems necessary to deduce optimal solutions, and only then starts investing in technology development.
This approach delivers unique advantages for developing B2B SaaS products to solve complex customer problems. While traditional methods might eventually reach the same destination through countless costly iterations, Deductive Innovation provides a map to the optimal solution before significant resources are committed. This radically reduces development waste and your risk of building the wrong product, shortens time-to-market for the simplest possible complete solution by years, and positions your product as the category leader from launch.
How Deductive Innovation Measures Optimality Objectively
The core of Deductive Innovation is a precise, objective approach to measuring Problem-Solution Fit: how well solutions solve customer problems. This addresses a fundamental gap in today’s methodologies: the inability to compare alternative solutions without building and testing them with customers.
In Deductive Innovation, a “customer problem” has a specific meaning: it describes a concrete situation where a particular target customer is trying to accomplish a valuable result. Expressed as one concrete example, a properly understood customer problem contains:
Starting point: What initiates the situation, when and why?
Desired end-result: What they are trying to accomplish in that situation, and why?
Circumstances: Aspects of the situation that cannot be changed by a solution, such as location, available resources, hard constraints, causalities and dependencies.
Evaluation criteria: How the customer judges both the end-result and the process of reaching it?
All these elements exist independently of any solution and can be discovered through systematic research. The customer’s own evaluation criteria are inherent parts of the problem itself, not subjective opinions of the solution developer.
With this understanding, we can objectively measure alternative solutions against two dimensions:
End-result quality: How good an end-result the solution enables customers to achieve, in principle, based on their own success criteria?
Process efficiency: How well customers can achieve that result, in practice? This is almost always defined in terms of speed, ease of use, and risk.
The primary measure is the number of actions the solution requires the customer to take to achieve the result, at a minimum. This can be objectively counted for any solution.
There are further more refined analytical measures e.g. for functional coverage (completeness of the solution), efficiency (proportion of required actions that are actually necessary), learnability (intuitiveness), reversibility (recovery from errors).
By counting actions across alternative solutions addressing the same problem, we often find order-of-magnitude differences that represent radical shifts in solution quality. This approach makes solution comparison objective and measurable without extensive user testing. The validity of the measurement depends on the validity of the customer problems.
Ultimately, Deductive Innovation allows us to predict which solution will create dramatically more value before investing in development, while empirical testing remains valuable for fine-tuning the details.
Most importantly, Deductive Innovation enables seeing where the market is headed long before competitors, giving us a first-mover advantage in creating the solution that will eventually dominate.
Breaking Through the Mental Barriers to Optimal Solutions
In the previous article, I identified five mental barriers that prevent product teams from pursuing optimal solutions. These deeply ingrained assumptions limit our ability to even ask the question “what is the best solution to this problem?”
Understanding these common barriers and how Deductive Innovation addresses them will give you valuable insight into why this approach is so powerful for developing B2B software products.
1) The Relativism Trap: “There are no best solutions”
The Relativism Trap, as described in part 1 of the series, is the common belief that there are no best solutions, making it pointless to seek them.
Deductive Innovation breaks through this trap with a simple mathematical fact: if we can compare alternative solutions, then a best solution necessarily exists.
To enable these comparisons, Deductive Innovation begins by discovering customer problems as objective facts in the world, independent of solutions. The criteria customers use to judge solutions are recognised as an inherent part of these problems.
While there aren’t “universally best” solutions that work in all possible contexts, specific situations definitely have optimal solutions. Different problems require different solutions, as I demonstrated in my earlier article by comparing alternatives for boiling water. The best solution depended on each specific situation.
As product developers, once we have discovered and chosen which problems to solve, we have the objective basis for comparison between alternative solutions.
2) The Measurement Challenge: “Only empirical testing produces reliable knowledge”
The Measurement Challenge refers to the difficulty of evaluating complex B2B solutions objectively as a whole, because testing them in real-life conditions is prohibitively expensive.
However, empirical testing isn’t the only way to acquire reliable knowledge. For example, we don’t need an unethical test to find out whether a cow would die if dropped from an airplane at 1000 metres. Why? Our understanding of the causes and effects that govern the situation gives us certainty without experimentation.
Similarly, instead of relying just on testing with customers, Deductive Evaluation measures solutions analytically against concrete instances of customer problems before anything is built. Because we know what a customer is trying to accomplish in a particular situation, we can measure how good a result a solution enables and what it requires the user to do. We obtain objective measurements with orders of magnitude less effort than empirical testing: hours instead of person-years for large solutions.
For example, imagine two solutions for planning delivery truck routes. One requires the user to manually try alternatives, and it takes 1218 actions to find just one feasible plan in a specific complex situation. The other uses an optimisation algorithm that incorporates customer’s criteria and constraints for good routes, evaluates millions of alternatives, and reduces delivery times by 10% with no user intervention. The superior solution is obvious without empirical testing.
This approach works best for:
functional needs (rather than emotional, aesthetic or social needs)
products used for their instrumental value (rather than intrinsic value)
large and complex customer problems
frequently-used products where customers value productivity
That makes Deductive Evaluation ideal for measuring and comparing B2B software solutions.
3) The Kilimanjaro Effect: All Solutions Appear Equally Good
In the first article, we used Mount Kilimanjaro to illustrate how existing solutions often appear equally good because they are all similarly far from optimal.
Deductive Evaluation provides two critical capabilities to overcome this effect:
First, it enables us to measure Problem-Solution Fit objectively, so we can map the “altitude” of the solution landscape around Kilimanjaro. Often, we indeed find that existing solutions are similarly mediocre, just in different ways – all lying on the low plains surrounding Kilimanjaro.
More importantly, we can measure how much better the Problem-Solution Fit could be without even designing a new solution. We can quantify potential improvement. While Deductive Design is required to actually locate the global maximum, if we find room for 9x, 12x, or 21x improvement, we know the highest peak is worth mapping.
The Kilimanjaro metaphor also shows how Deductive Innovation and iterative methods complement each other. Deductive Innovation identifies Kilimanjaro (the global optimum), while iteration can help climb to the peak once you’re on the right mountain.
4) The Cost Fallacy: “It costs more to build a better solution”
As discussed in the first article, the Cost Fallacy assumes superior solutions cost more to build, a misconception that is particularly pronounced in B2B software.
Deductive Evaluation exposes why this fallacy doesn’t hold: When evaluation reveals that an optimal solution would require 10x fewer user actions than existing alternatives, it implies current solutions contain enormous unnecessary complexity. This customer-facing complexity inevitably creates unnecessary internal technical complexity, often compounded by years of iteration and accumulated architectural debt.
The optimal solution is always the simplest one that fully addresses the complete customer problem. Deductive Innovation systematically identifies this simplest solution, eliminating unnecessary complexity from both the customer’s perspective and the technical implementation.
By pursuing optimality from the start, we can simultaneously reduce development costs, speed up time-to-market of an optimal solution, and increase customer value – turning the traditional “good, fast, cheap: pick two” constraint on its head.
5) The Impermanence Fallacy: “Customer needs change fast and we must adapt”
The Impermanence Fallacy, as we explored in the previous article, is the myth that customer needs change rapidly. This stems from confusing solutions with problems. While solutions evolve quickly with technology, the underlying customer problems remain remarkably stable.
Deductive Innovation addresses this fallacy by:
Providing a novel definition of what “customer problems” are, in principle, enabling companies to distinguish enduring problems from evolving solutions
Offering methods to discover these stable problems systematically
Building long-term product strategy on this stable foundation
Understanding this stability gives you the confidence to make substantial investments in understanding customer problems deeply. As technology evolves faster than ever, this deep problem knowledge becomes more valuable than ever. As late Steve Jobs famously said:4
You’ve got to start with the customer experience and work backwards to the technology. You can’t start with the technology and try to figure out where you’re going to try to sell it.
Deductive Innovation gives you a systematic way to follow this advice, allowing you to work backward from stable customer problems to ideal solutions.
Rather than chasing technological trends, companies adapt new technologies to solve customer problems, and create optimal solutions that remain relevant for extended periods despite technological change.
Addressing Six Key Concerns About Deductive Innovation
Given how much Deductive Innovation differs from today’s best practices, it usually raises important questions and concerns that prevent experienced software professionals from embracing it.
1. “Isn’t This Just Waterfall Development?”
When I describe Deductive Innovation’s emphasis on discovering problems before designing solutions and defining the optimal solution before building it, almost all competent software professionals immediately ask: “Isn’t this just waterfall development with a new name?”
This concern is most understandable. The waterfall model’s notorious failures led to the agile revolution. But Deductive Innovation differs from traditional waterfall development in critical ways.
Waterfall asked customers to provide “requirements” – essentially solution descriptions – which they simply cannot reliably do. There’s no way to validate if such requirements are correct or complete until after building the solution. In countless cases when the customers finally got their hands on the software, the solution turned out problematic in multiple ways, if not a complete failure. This often happened 1-3 years after development started.
In contrast, Deductive Innovation starts the whole software process with something customers can genuinely validate: their problems, expressed as concrete situations that occur in their world. Customers can easily recognise if our description of their problems and situations matches their reality. We can validate this understanding even before any thought is spent on solutions.
As long as the customer problems we have mapped are valid and described in a way that enables deducing the solution, correct application of the deductive process will produce a good solution that is likely to impress customers when we validate it with them.
2. “How Can You Evaluate Without Customer Testing?”
Another common concern is: “How can you evaluate solutions without extensive customer testing? Aren’t customers the ultimate judges of any product?”
This question gets to the heart of the Measurement Challenge we discussed earlier. For B2B software that serves functional needs, we can analyse how well a solution addresses specific aspects of customer problems without building the product.
The key is having a sufficiently detailed understanding of the customer problem expressed as a particular instance of a real-life situation, including the concrete circumstances, stakeholders involved, goals, desired outcomes, and evaluation criteria. With this understanding, we can reliably predict how specific aspects of the solution will impact the use of the product and the value it creates for customers.
3. “This Limits Creativity”
“But what about real innovation? Doesn’t this approach limit creativity and breakthrough thinking?”
This concern misunderstands the relationship between constraints and creativity. Deductive Innovation enhances innovation by focusing creative energy on solving the problems that are actually novel and unique, instead of using ideation to come up with suboptimal solutions to recurring problems that can be solved directly by deduction.
I like to compare this to mathematics. You could solve a group of linear equations by guessing and iterating, but an analytical method, Gaussian elimination, gives you the right answer straight away. But you certainly need lots of creativity and new ideas to solve unsolved unique problems, like proving the Riemann Hypothesis.
The most innovative products aren’t random strokes of genius but precise answers to well-defined problems. By deeply understanding customer problems, you create a fertile ground for meaningful innovation that delivers actual value rather than novelty for its own sake.
4. “This Doesn’t Work for Emerging Technologies like AI”
“This might work for established markets, but what about emerging technologies where customer problems aren’t well-defined yet, like generative AI or AI agents?”
Even with emerging technologies, customer problems exist before solutions. When cloud computing emerged, the fundamental problems businesses faced (like managing IT infrastructure, scaling computing resources efficiently, reducing capital expenditure) hadn’t changed. The technology simply offered a better solution.
By identifying which stable customer problems a new technology could address more optimally, you can predict its eventual application and impact.
5. “This Slows Us Down in a Fast-Moving Market”
“But what about fast-moving markets where speed is critical? Won’t this approach slow us down?”
This is a common misconception. While Deductive Innovation requires a small team of 1-3 people to spend a few weeks or months for upfront problem discovery, it dramatically accelerates overall time-to-market for complete solutions by minimising costly wrong turns, eliminating major pivots, and preventing accumulation of unnecessary complexity. This creates an extremely favorable return on investment: a small, focused effort that prevents the much larger waste of building the wrong product or refining a suboptimal solution.
In truly fast-moving markets, where companies rapidly develop better solutions to existing problems, those with deep customer understanding actually move faster because they aren’t wasting resources on incremental improvements or solving the wrong problems entirely.
Being first is also vastly overrated compared to being significantly better. Market leaders like Apple, Zoom, Figma, HubSpot and Shopify weren’t first movers – they were better movers who entered established markets with superior solutions that redefined customer expectations. Their success came not from rushing to market but from deeply understanding what customers truly needed and delivering solutions with dramatically better Problem-Solution Fit.
6. “This Leads to Analysis-Paralysis”
There is a crucial distinction between deep problem analysis and analysis-paralysis. Analysis-paralysis occurs when teams get stuck in research without being able to make decisions. This often happens because they lack clear criteria for what information to discover and what constitutes “enough” understanding.
Deductive Innovation specifically addresses these root causes. We conduct focused, bounded discovery with a clear purpose: gathering precisely the information needed to understand customer situations and deduce effective solutions. This research has well-defined completion criteria: we have gathered enough when we can measure complete solutions analytically for specific customer situations. Unlike open-ended exploration, the discovery process targets only elements directly relevant to designing solutions.
When I use terms like “optimal” and “best,” I mean them aspirationally, not literally. In markets where existing solutions are far from optimal, finding a solution that’s 80% of the way to perfection creates transformative 10x improvements. Given that 10x represents a 1000% improvement, the last 20% matters little compared to identifying the right mountain to climb in the first place. Once the solution is on the right mountain, iteration works fine to refine the product.
Deductive Innovation includes built-in safeguards against actual paralysis. We can validate our problem understanding with customers before solution design, validate our solution concepts before development, and build incrementally once we have identified the right destination. Rather than paralysing progress, this sequential risk-reduction approach accelerates time-to-market for truly valuable solutions by eliminating expensive rework.
These clarifications help distinguish Deductive Innovation from both traditional waterfall development and iterative approaches. To make these distinctions even clearer, let’s compare it directly with today’s best practices.
Comparison With Today’s Best Practices
To better understand how Deductive Innovation differs from conventional product development practices, consider the comparison table below.
This comparison highlights why Deductive Innovation is particularly valuable in B2B and Enterprise SaaS contexts where existing solutions are far from optimal. While traditional methods excel at incremental improvements, they are less equipped to identify transformative opportunities in a complex problem space.
Next: From Theory to Practical Examples
In the next article, we will move from theory to practice. I will share real examples of major market predictions that proved accurate years in advance – not through luck or genius, but through systematic analysis. You will also learn practical steps to developing your own market prediction capabilities and validating your solutions without expensive trial and error.
This is the second part of a 4-part series on market evolution and how to predict it using Deductive Innovation. Continue to Part 3 of the series:
Three Cases of Predicting Market Evolution to 10x Optimal Solutions Years in Advance
Discover how analysing and reasoning about customer problems enabled predicting major market shifts years in advance, and how you can develop this same competitive advantage to 10x your B2B SaaS.
This is the third article in a 4-part series on predicting market evolution in B2B SaaS.
Subscribe now to be notified when the next article is published, and receive your free report “The Secrets of Gaining an Unfair Advantage for B2B SaaS Products” packed with insights from in-depth interviews with 31 B2B SaaS product executives and founders:
Ken Beck et al: “Manifesto for Agile Software Development”, 2001, https://agilemanifesto.org
Peter Thiel with Blake Masters: “Zero to One: Notes on Startups, or How to Build the Future”, Crown Business, 2014
Image shows the incorrect medieval understanding of trajectories based on Aristotle’s flawed theory of motion. Image source: Janet Heine Barnett (2009): “Mathematics goes ballistic: Benjamin Robins, Leonhard Euler, and the mathematical education of military engineers”, BSHM Bulletin: Journal of the British Society for the History of Mathematics, 24:2, 92-104. Cited in: Julian Estevez (2019): “Book Review: The study of parabolic shooting without computers”, 22 Feb 2019, https://www.dyna-energia.com/book-review/the-study-of-parabolic-shooting-without-computers
Steve Jobs in an answer to a question from the floor during the keynote at Apple’s Worldwide Developers Conference in 1997. YouTube video: https://www.youtube.com/watch?v=oeqPrUmVz-o