Three Cases of Predicting Market Evolution to 10x Optimal Solutions Years in Advance (Part 3)
Discover how analysing and reasoning about customer problems enabled predicting major market shifts years in advance, and how you can develop this same competitive advantage to 10x your B2B SaaS.
👋 Hi, it’s Antti LK and this is the third article in a 4-part series on predicting market evolution in B2B SaaS. Read Part 1 and Part 2 if you haven’t already. And subscribe now to make sure you don’t miss the next articles!
Predicting market evolution isn’t just theoretical speculation. It’s a skill that can be systematically developed and applied. Many product developers who focus on finding optimal solutions have observed how markets consistently converge toward these solutions, making it possible to anticipate major shifts years before they materialise.
In this article, we will explore concrete examples of how understanding customer problems and reasoning through their best solutions can lead to accurately anticipating market direction years in advance. We will also see how you can validate your own predictions efficiently without costly trial and error.
For readers just joining this series: The first two articles established how markets converge toward optimal solutions and discussed Deductive Innovation, a systematic methodology to understand customer problems and identify such best solutions before competitors. The key to predicting where the market is going is answering the question: “What is the best solution to this customer problem?”
At the core of market prediction lies the concept of “10x solutions”: revolutionary advancements that outperform existing alternatives by an order of magnitude or more. As Peter Thiel noted in Zero to One:1
Once you’re 10x better, you escape competition.
When we understand customer problems correctly, we can systematically identify these 10x opportunities years before competitors. If a solution could be 10x better, a competitive market will ensure that it will emerge, whether we create it or let someone else win.
Market Evolution in Action: When Industries Inevitably Found Optimal Solutions
During my career in product development, I’ve repeatedly seen how analysing customer problems can reveal future market directions with surprising clarity – not through any special insight, but through analysing customer problems and reasoning their optimal solutions.
What follows are three stories from the early part of my professional journey where our design team identified the direction of market evolution years before it happened. These examples aren’t tales of lucky guesses. They demonstrate how logical analysis can anticipate future developments, even when established industry players miss these opportunities. Sometimes this led to the frustration of watching others eventually implement solutions we had identified earlier.
Among various experiences with this pattern, these three cases were particularly formative for me. They helped establish my conviction that markets tend to follow predictable paths toward optimality that can be mapped in advance. They were foundational inspiration for my passion to uncover the principles of systematic product innovation that can help companies gain first-mover advantages.
1) The Touchscreen Prophecy: The Inevitable Evolution of Screens in Mobile Computing
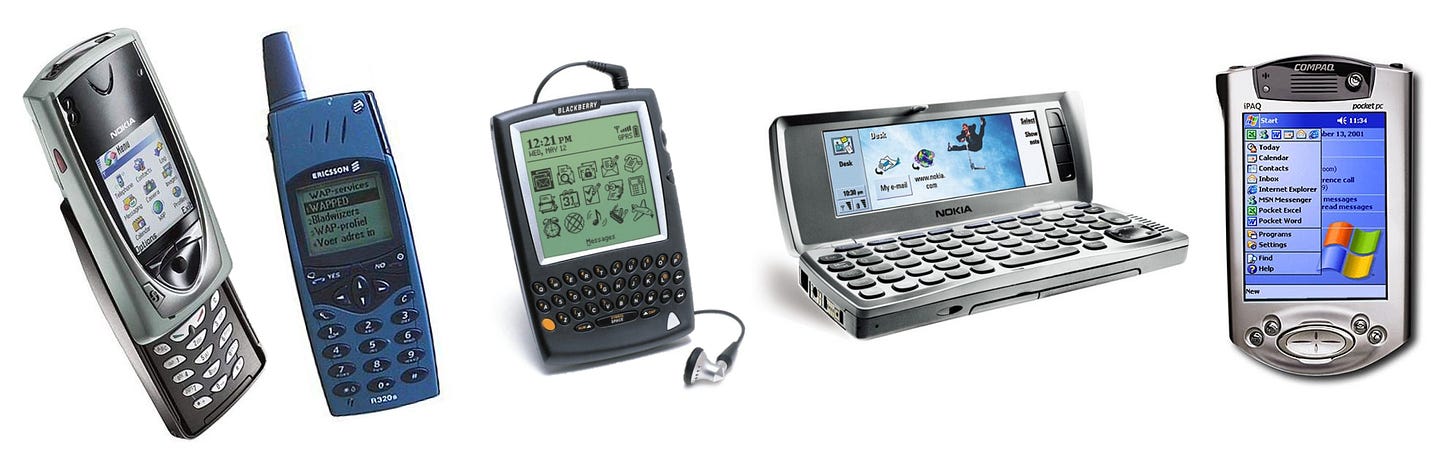
By the year 2000, many people in the Finnish tech industry recognised that mobile internet would become reality, though the optimal form factor remained an open question. To some of us, it seemed evident both theoretically and practically that an effective internet-connected pocket computer would need a large touchscreen.
Why a touchscreen? The case for touchscreens was grounded in established interface design principles, many of which I had covered in my thesis work. Direct manipulation had been recognised as effective for user interfaces since the 1980s.2 Although desktop graphical interfaces claimed to provide “direct” manipulation, users were actually guiding a pointer indirectly by moving a mouse. While large vertical computer monitors were not practical for touchscreens (your arm gets tired), for handheld devices they offered truly direct interaction making them potentially superior if the technology could work reliably.
Why a large screen? In early 2001, I worked in a Nokia-commissioned project to design a personalised news portal for WAP phones (early smartphones). The experience highlighted the limitations of devices that could display only 2-4 lines of text. Using these early internet-capable phones was challenging: waiting ages for sites to load, squinting at cramped text on tiny monochrome screens, and constantly scrolling after every 1-2 sentences. It was like trying to read a novel through a keyhole. This led our design team to conclude that effective internet browsing on mobile devices would require much larger screens.
Nokia and many other established mobile phone manufacturers continued pursuing different approaches. When Apple released the iPhone in 2007, it represented the commercial realisation of what many interface designers had envisioned: a device centred around a large touchscreen optimised for internet use.
The market quickly converged on the optimal solution: internet-capable pocket computers with large touchscreens. Other types of phones and pocket devices essentially disappeared.
The prediction was a logical result based on understanding the situations that mobile users encountered, where accessing and interacting with internet content would help them while away from their desks. The optimal solution could be anticipated years in advance by anyone who understood the problem space and potential alternative solutions.
The 10x advantage of touchscreens became apparent soon after the iPhone’s release. The interface was so intuitive – tapping directly on objects, swiping to navigate, pinching to zoom – that it reduced the learning threshold dramatically. In 2010 when my nephew was just 18 months old, he eagerly used my iPhone to draw and play simple games with remarkable ease. Videos of monkeys browsing Instagram on iPhones demonstrate how the direct interaction eliminated countless hurdles that animals couldn’t overcome in previous interfaces. The large responsive touchscreen with pinch-to-zoom gesture enabled the iPhone’s browser to provide a desktop-like browsing experience, and websites designed for desktop screens became usable on mobile for the first time. Consequently mobile data usage exploded and forced US mobile operators to change their business models.3
2) The Instant Search Revelation: Foreseeing the Evolution of Desktop Search
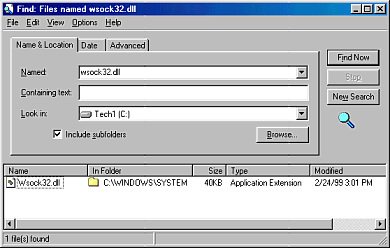
Around 2001, the limitations of Windows file search were painfully apparent to anyone who used it. A typical search scenario involved typing part of a filename, clicking the misleadingly labelled Find Now, and then watching a magnifying glass sweep back and forth for minutes, often ending with no results even when the file definitely existed.
Meanwhile, Google was returning results from the entire internet in less than a second. The contrast was infuriating, like comparing a horse-drawn carriage to a Ferrari. The experience was so consistently terrible that many people avoided using Windows search entirely. Instead, they maintained elaborate folder hierarchies and painstakingly browsed through them.
Recognising this problem, my designer colleague Karri-Pekka Laakso decided to actually build a proper solution. The optimal approach required indexing all files on a PC to provide search results immediately as the user typing. He implemented a fast algorithm that supported substring search where the search string could appear anywhere in the filename, not just at the beginning of a word. No wild cards needed. His working prototype (see image below) demonstrated how dramatically better the user experience could be with this approach.
Neither Microsoft nor other Windows software providers implemented this superior solution at the time. Then at Apple’s WWDC in June 2004, Steve Jobs lamented how “it’s easier to find something from one of a billion websites on the web with Google than it is to find something on your hard disk,” and proceeded to demonstrate Apple’s solution, Spotlight.4 This validated the obvious improvement opportunity in desktop computing.
Over time, all operating systems implemented a fast system-wide search. The improvement was radical: what once took minutes now took milliseconds, a 100-1000x speed improvement. Tasks that were so frustrating users avoided them entirely became effortless background activities.
These examples reveal a pattern: When optimal solutions offer over 10x improvements, markets eventually converge toward them and fundamentally change user behavior. Market evolution becomes predictable when viewed through the lens of optimal solutions to customer problems. Desktop search evolution was convergence towards the solution users obviously needed all along.
3) The App Store Prophecy: Predicting Apple’s $30B Business Six Years Early
In 2002, Nokia tasked our design consultancy with designing a technical solution for installing apps on smartphones that would be both secure and user-friendly.
Nokia’s Communicator smartphones used the same approach as Windows: browse to a developer’s website, click through confusing security warnings, download an installation package, run it, navigate through a multi-step installation wizard with cryptic technical options, and finally find the app on your desktop. The process was tedious and unsecure even for technical users. Apps could contain malware, and criminals could impersonate legitimate companies. Most users simply clicked “Yes” to every prompt without reading, just to make the interruptions stop.
Digital certificates existed so developers could sign their apps, but users didn’t understand these concepts and simply ignored them. Even if users checked certificates, what would that help with less known software developers?
Our 2002 report noted: “[Today] the easiest way to operate the system is often the insecure way. Turning this the other way round … is challenging, but it can be done. Realising such a secure and usable environment is the responsibility of the [manufacturer].”
Instead of limiting the analysis to purely technical solutions, our approach focused on identifying the best possible solution by reasoning from first principles.
The analysis concluded that no purely technical solution could make app installation both secure and easy. Instead, we proposed combining technology with a centralised distribution model: “A much more fruitful approach is to combine judicial and financial incentives... Since users necessarily trust their device manufacturer, the manufacturer is the most natural party to take responsibility for security.”
The manufacturer would:
Verify developers’ identities and establish contracts with financial penalties for malicious behavior
Test software with standard security tests before distribution
Handle updates centrally rather than allowing apps to update themselves
Possibly inspect and certify free apps at no cost, “since popular software is likely to improve the device’s market position.”
Using this model “software can always be installed without any [security] questions or output to the user … [This follows] the principle of making the easiest way to achieve a goal also the safest way.”
Nokia did not implement these recommendations. Like many market leaders, they focused on incremental improvements to maintain their position.
When Apple announced the App Store six years later in 2008, it validated the central distribution model approach. The market quickly converged on this solution: third-party apps are distributed centrally by the device manufacturer or operating system developer.
This wasn’t a matter of lucky prediction. It demonstrated how analysing core problems (in this case, secure and easy app installation) and systematically reasoning toward optimal solutions can reveal where markets will eventually move.
The 10x advantage of the App Store was apparent in multiple dimensions. It reduced the process of finding and installing an app from several minutes and dozens of steps to a few simple taps that took seconds. The most dramatic 10x improvement came in security. The traditional software installation model led to widespread distribution of viruses and malware on the Windows platform. By contrast, Apple’s App Store has experienced remarkably few security incidents. This risk reduction by orders of magnitude is another example of how the best solutions completely reshape the experience.
The introduction of the App Store highlighted an important lesson beyond just predicting market direction: Seeing optimal solutions isn’t enough. To create the future, organisations need a culture focused on identifying the best solutions and a commitment to implementing them. It's noteworthy that in all three examples discussed, Apple was the company that introduced optimal 10x solutions to the market. Their culture of pursuing excellence rather than incremental improvements is likely a key factor in this pattern.
Today, Apple’s App Store generates over $30 billion annually with an estimated 85% operating margin.5 Apple implemented Peter Thiel’s advice we discussed in the previous article: it looked for the global optimum, found it, and created a monopolistic advantage for itself. The App Store as a stand-alone business now exceeds the size and value of Nokia’s entire mobile phone business at its 2007 peak6, the year before the App Store launched.
These three cases illustrate how markets tend to converge toward solutions that address customer problems with minimal complexity.
But prediction isn’t perfect, especially when fundamentally new technologies emerge that change what’s possible. Let me share a case where the crystal ball didn’t work.
Prediction Meets Technological Disruption: A Cautionary Tale
While I have shared examples of how analysing customer problems can lead to accurate anticipation of market evolution, intellectual honesty requires acknowledging the limitations of this approach. One significant area where this predictive method fell short was in anticipating how natural language search would evolve.
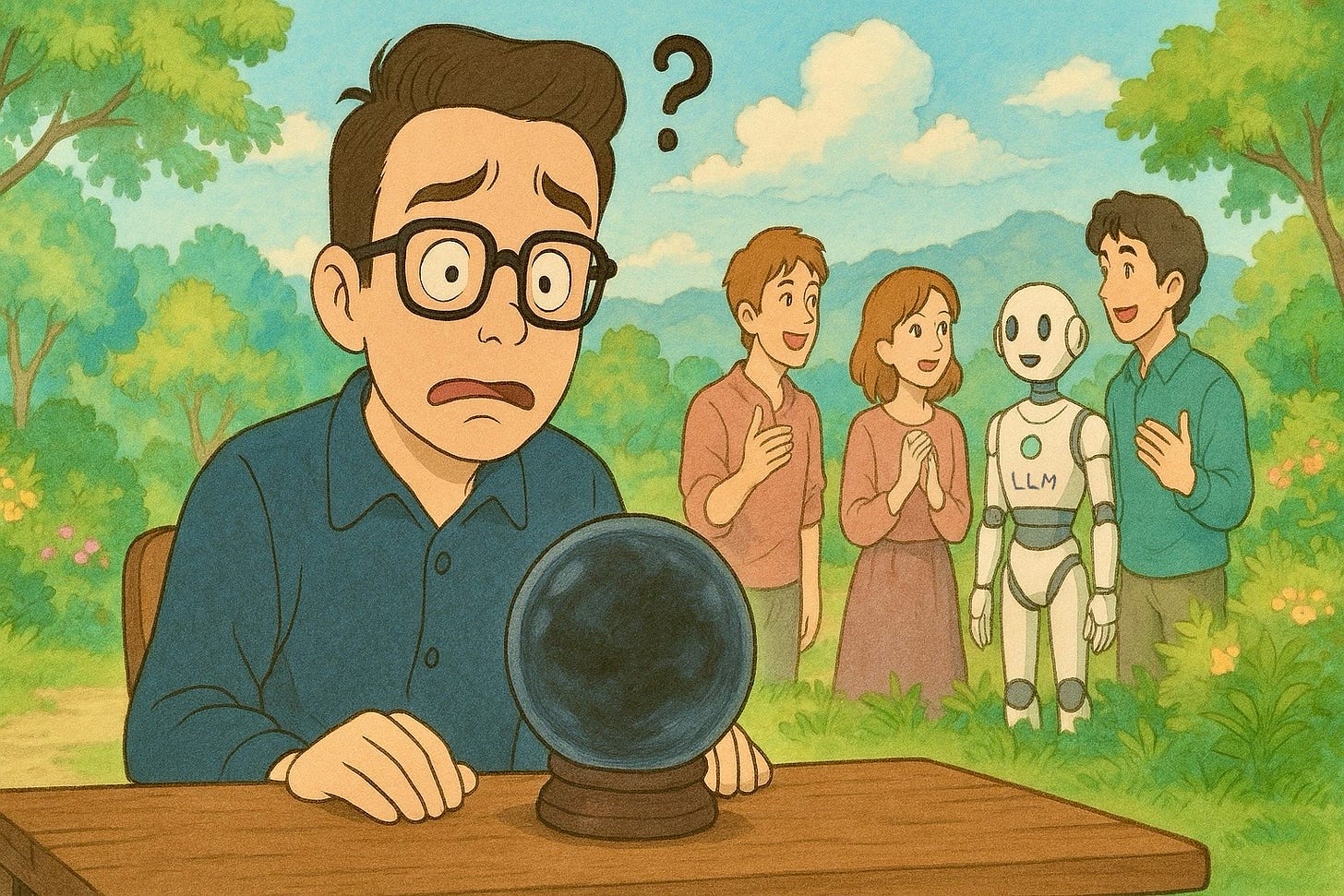
For years, I advised against natural language search interfaces. I recommended focusing on precise filtering capabilities, logical and consistent filter behaviour, search result organisation, visual representation of how filters affect results, and informative result previews. I considered these approaches optimal because they gave users explicit control while minimising the ambiguity inherent in natural language.
When asked about “smart search” or conversational search interfaces, I would explain why they weren’t practical: machines couldn’t truly understand language, handle ambiguity, or provide contextually appropriate responses without extensive programming for each possible scenario.
When ChatGPT and other LLMs emerged they fundamentally changed what was possible. Suddenly, users could type natural language questions and receive remarkably accurate, contextually appropriate responses. The idea I had dismissed as impractical became not just viable but transformative almost overnight.
This example demonstrates an important limitation of the Deductive Innovation approach: it works well for predicting how existing technologies will be optimally integrated and applied to solve customer problems, but it cannot predict entirely new technologies that change what’s possible.
Interestingly, while I failed to predict this technological breakthrough, the principle of imagining optimal solutions still holds value. Science fiction writers had long envisioned computers that could understand and respond to natural language as the ideal interface. They recognised this as the optimal solution decades before it became technically feasible. In a sense, they applied the same principle: they imagined the ideal solution, but regardless of current limitations of technology.7
This limitation reinforces an important aspect of market prediction: we must separate predicting how existing technologies will be optimally applied (where systematic analysis can provide clear insights and where opportunities abound) from predicting technological breakthroughs themselves, which is and will remain unpredictable.8 The most dramatic market shifts often occur when a new technology suddenly makes previously “impossible” solutions possible.
While Deductive Innovation provides foresight on the use of existing technologies, we must remain humble about the potential for technological disruption to change what “optimal” means overnight.
The Challenge of Discovering Customer Problems
Even though technological breakthroughs like LLMs can disrupt market predictions, they represent the exception rather than the rule. Most market evolution follows predictable paths based on solving existing customer problems with existing technologies.
In these cases, the key to identifying optimal solutions and thus anticipating market direction lies in understanding customer problems deeply. This is unfortunately one of the most difficult and least understood aspects of the entire process. This challenge explains why many product teams resort to collecting feature requests or addressing surface-level pain points, rather than investing in discovering the underlying problems customers are trying to solve.
What Customer Problems Really Are
What makes customer problem discovery so difficult?
First, customers themselves rarely articulate their needs independently of solutions. They express needs as feature requests (“we need a dashboard”), pain points (“this process is so complicated”), or complaints about current solutions .
Second, the whole industry lacks clarity about what “customer problems” are, even in principle. Without a clear definition, it’s impossible to systematically discover and analyse them.
This conceptual gap directly impacts market prediction ability. Companies that only understand surface symptoms will create incremental improvements to existing solutions, while those who grasp underlying problems can envision entirely new, 10x solutions that will eventually dominate markets. The difference between these approaches is the difference between following market trends and leading them.
The Deductive Innovation approach tackles these challenges by offering a specific definition of customer problems. To recap, this definition answers the question: “What in principle is that customer problem to which a software product, as a whole, is a solution?” That kind of a customer problem describes a concrete real-world situation where a particular customer is trying to accomplish something valuable.
When we understand customer problems correctly, we can:
Distinguish between genuinely different customer problems that require a different optimal solution
Recognise which problems are common across different customers and are the basis for developing a common solution (a product)
Separate passing fads and trends from the fundamental customer needs
Identify which problems, if solved optimally, would create the most value
When we conduct problem discovery, the definition helps distinguish between very different kinds of problems:
Customer pain points, which are problems in the current solution, not the problem to which the solution is a suboptimal one
Features, which frustrated customers ask for, but which are themselves solutions, not customer problems
Fundamental customer problems, which exist independently of any solution
The definition differs significantly from how customer problems are commonly understood in product development. When I ask an LLM to give me examples of customer problems in the context of today’s best practices (like lean startup or service design), it typically provides examples of either pain points or features that customers “need”, neither of which are customer problems in the sense that Deductive Innovation defines the term.
Deductive Discovery provides a systematic methodology to uncover these customer problems. The methodology relies on contextual interviews, starting with executives and managers, and proceeds to observing the practical work of end-users. For market prediction purposes, what’s crucial is that it helps us identify problems that exist regardless of current solutions, and will therefore drive market evolution over time. This approach lets us see where markets must eventually go, not because of technological trends, but because of stable customer needs.
Using Customer Input as Clues to Deeper Problems
To illustrate the distinction between different kinds of “problems”, consider this real B2B example.
A customer of a tool for designing telecommunications service configurations contacted the company where I worked and was clearly frustrated:
“Importing and exporting the configurations is too slow, it takes hours,” they complained (customer pain).
“We need a fast import and export,” they demanded (feature request)..
They made it clear this wasn’t a minor inconvenience but something that was destroying their team's productivity.
The customer clearly had a problem of some kind, and obviously something was wrong with the existing solution. But neither of these statements are that kind of customer problem to which the slow import and export features were a bad solution. Input like this from customers is important to acknowledge, but must not be taken at face value. It is just a clue about where the real issue is, and acts as a starting point for problem discovery.
Through deeper investigation, the actual situation where the issues arose became clear: The customer was developing new technical configurations for a large number of telco services, requiring thousands of changes to the configuration data. The existing tool was so cumbersome that making just one change easily required dozens of clicks.
As a workaround, they had exported all the data to Excel, made edits there because it was faster, and then imported the data back. Each import and export took a couple of hours. Because they were still in the design stage, they needed to test how the service configurations worked, and then edit again based on the results. One iteration of the whole process took many hours, easily as much as a full day, which was a major pain in the neck.
Engineers would often start import processes at the end of the day, returning in the morning hoping to find successful results rather than error messages. Due to complex interdependencies in the configuration data, their Excel workaround couldn’t enforce the validity of the configurations, so often the import process would end in failure, leading to hours of wasted effort. The existing process wasn’t just inefficient, it was affecting project timelines and undermining morale.
Quantifying the Impact of Solving the Real Problem
The optimal solution was not simply a better import and export feature.
What was needed was a tool with which customers could actually design large changes to technical configurations and test them efficiently in real situations. The potential impact could be quantified – another example of the 10x pattern that characterises transformative solutions:
Interface efficiency: Instead of dozens of clicks for one change, an optimal tool would require just a couple of clicks – roughly a factor 10x reduction.
Batch operations: An optimal tool would enable making the same change to multiple configurations simultaneously, providing another factor 10x reduction in effort, for a ~100x total reduction compared to the existing tool.
Validation speed: The Excel workaround required a 2-3-hour import process to find out if there were validation errors. The optimal tool would either prevent invalid edits or highlight them immediately, reducing feedback time from hours to seconds, for another improvement of more than 100x.
These improvements would make the overall process at least 10x more efficient than either the original tool or the workaround solution. This magnitude of improvement is what enables a solution to reshape the market.
From Problem Understanding to Market Prediction
This little example illustrates a critical principle of market prediction: companies that identify and solve fundamental customer problems optimally will eventually outcompete those who spend their development resources merely addressing symptoms.
Companies that focus only on addressing immediate pain points through workarounds like faster import/export might gain short-term advantages. However, organisations that recognise and address the actual underlying problem can create solutions with orders of magnitude better Problem-Solution Fit. Over time, these 10x and 100x improvements inevitably attract customers and define the direction of market evolution.
This pattern repeats across software markets. The companies that lead markets are those who look beyond feature requests and pain points. They discover the underlying customer problems, seek the best possible solutions, and develop radically better products.
Understanding the real customer problem – the real-life situation where specific customers are trying to achieve an outcome they need – enables teams to find optimal solutions that others might miss. This understanding doesn’t improve just product development. It also informs market positioning by revealing the direction in which the market will likely evolve.
With a deep understanding of customer problems, teams can anticipate market direction, and in addition, validate their vision before committing significant resources.
Validation in Practice: Reducing Risk Without Excessive Iteration
Building on the telco configuration example, let’s examine how validation works in the Deductive Innovation approach.
When I discuss how this approach enables comparing solutions analytically without customer testing, and defining optimal solutions without iterating with customers, a common concern relates to customer validation.
Since customers are the ultimate judges of any product, how can we be confident in solutions defined through deduction? This concern deserves careful attention.
The good news is that validation doesn’t require building and iterating on complete products. Deductive Innovation incorporates validation at multiple strategic points in the development process. This dramatically reduces risk and avoids the cycles of build-measure-learn that characterise many zero-to-one product development efforts.
A Multi-Level Validation Approach
Deductive Innovation employs a two-stage validation process that addresses risks at each critical juncture:
1. Problem Validation
Before defining any solution, we validate our understanding of customer problems across multiple customers to ensure accuracy, sufficient completeness, and commonality. This moves the first validation to the earliest possible stage of product development.
While approaches like lean startup and design thinking also emphasise validating “problems” before solutions, they tend to focus on validating pain points or gaps in current solutions. Deductive Innovation validates target customers’ real-world situations – elements like their goals, concrete circumstances, desired end-results, causal dependencies, constraints, and evaluation criteria.
Customers can easily confirm whether our descriptions match their reality because we are discussing their world as it exists today, not some hypothetical future.
In the telco example, problem validation wouldn’t just confirm that “importing and exporting takes too long” (a pain point). Instead, we would validate across a few customers that they regularly face situations where they need to configure many services simultaneously. By confirming that this demanding situation occurs across customers, we validate that solving it would create significant customer value.
2. Solution Validation
Once we have designed an optimal solution, we validate the solution progressively with customers before implementation through:
Scenario-based storytelling: Narratives that show how customers would use the solution to solve specific real-world problems (start with these)
Storyboards: Visual step-by-step illustrations showing the solution in action
Low-fidelity prototypes: Clickthrough user interface mockups with realistic example data that show the end-to-end interaction flow for the most important situations (most recommended)
High-fidelity prototypes: Clickable throw-away prototypes that implement key logic for the core parts of the solution (use these only if necessary to demonstrate complicated logic)
Unlike abstract requirement documents, customers can reliably evaluate these tangible representations that show how the solution works in their real situations. For our telco example, solution validation would involve walking a customer through a mockup of a specific complex configuration scenario.
A solution designed using Deductive Design solves a previously validated concrete problem very directly. It is almost certain to resonate well with customers, unlike solutions built on assumptions. At this point, we usually need to pay attention just to catching minor details of the customer’s situation that we may have initially missed.
Success Stories of Market Leadership Through Early Validation
This kind of validation approach has proven effective in positioning companies as frontrunners before significant development resources are committed. Here are two examples that demonstrate how early validation of optimal solutions can secure competitive advantages.
In one case, during a competitive bid with a leading European telecommunications company where we were falling behind, my team conducted two months of Deductive Discovery with the prospect. After mapping their relevant problems comprehensively, we designed an optimal product concept based on that understanding.
When we presented our low-fidelity mockup and demonstrated how it addressed their needs, the prospect was visibly impressed by our deep understanding of their problems and a clear vision for a solution that exceeded their expectations. According to our manager who worked with the customer, this turned the tide at the last minute and secured a €5M deal we nearly lost – all based on “just” a mockup and a plan.
This wasn’t just about closing a single deal. The approach positioned our company as a thought leader by identifying valuable customer problems that informed the whole product suite. By deeply understanding the challenges in a telecommunications niche and designing an optimal solution, we distinguished ourselves as innovators rather than followers – all before writing a single line of code.
Another example comes from a founder I interviewed who followed a similar approach. They first researched customer needs by interviewing about 20 potential customers, then devised a solution based on their discovery. When they presented their solution concept to an early prospect, the customer immediately recognised its value: “This is so good that you should set up a new company. We’ll cover the costs upfront and act as a reference customer.” The founder added with amusement: “They basically pitched it back to us in a way that it would’ve been foolish to say no.”
This founder didn’t just make a sale. They established a strong competitive position from day one. Their solution was so clearly superior to existing alternatives that customers were willing to fund its development. This first-mover advantage gave them a significant head start over competitors who were still developing incremental improvements to existing products.
These examples demonstrate a critical aspect of effective product development: when an optimal solution addresses a validated customer problem, market validation often comes swiftly. Customers recognise the value immediately because the solution aligns perfectly with problems they’ve been struggling to solve.
The Validation Advantage
This validation approach offers several advantages over traditional methods:
Early risk reduction: Major risks are identified at the earliest possible stage before committing significant development resources
Reliable feedback: Customers first validate problems, and later evaluate solutions in the context of their validated problems.
Faster time-to-value: No time is spent building features that don’t address real validated customer problems
Focused development: Engineering teams work from a validated foundation rather than hypotheses
By validating both customer problems and solutions before major implementation, Deductive Innovation dramatically reduces the risk of building products customers won’t buy while avoiding the expensive and time-consuming cycle of building, measuring, and learning that characterises today’s best practices.
The validation advantage isn’t just about reducing risk. It’s about establishing market leadership by being the first to identify and deliver the optimal solution that the market will eventually converge toward.
Looking Ahead: Where Will the B2B SaaS Market Move Next?
The examples above demonstrate a repeatable pattern that can help identify where markets are heading. Based on the principles discussed, here is a specific prediction for B2B SaaS markets I’ve been making since 2018:
For enterprise software, the optimal solution is an integrated platform-level solution that covers all common customer needs within one specific industry vertical.
Imagine a conference hotel chain, a residential construction firm, or a veterinary clinic. Today these businesses often rely on 20 to 100+ different software tools to operate effectively. Many are generic, industry-agnostic platforms. Others are custom-built solutions or scattered Excel spreadsheets. As a result, they must invest significant time and resources into assembling, integrating, configuring, and maintaining a fragmented IT stack.
Customers don’t want to piece together solutions. The future lies in well-integrated platforms purpose-built for specific industry niches that address virtually all customer problems in one place. The core product strategy in enterprise software should always focus on solving customers’ overall problem within a single, holistic platform that is designed as one integrated whole.
The 10x advantage of vertical platforms comes from consolidating core functionality and data, eliminating integration headaches, providing consistent interfaces, and automating cross-functional workflows that previously required manual steps across multiple systems. For businesses, this means drastically reduced IT complexity and thousands of saved hours annually. For employees, it means 10x fewer logins, interfaces to learn, and context switches throughout their day.
This market evolution has already started to play out. In the US, companies like Toast (restaurants), ServiceTitan (home services) and Veeva (life sciences) demonstrate how vertical-specific platforms can dominate their industries by addressing comprehensive sets of customer problems in an integrated way.9
This perspective is gaining increasing traction among the industry. In August 2023, Thiel Fellow Luke Sophinos predicted a “vertical SaaS tsunami” that will transform all industries.10
Many of the most successful B2B SaaS companies of the next decade will
identify an industry with suboptimal software solutions,
deeply understand their complete problem space, and
build integrated vertical platforms that address the problems holistically, rather than competing feature-by-feature with established horizontal solutions.
Next: Your Framework for Market Prediction
What these examples demonstrate is that anticipating market evolution isn’t about spotting minor improvements or following trends. It’s about understanding customer problems deeply and asking: “What would be the best solution for this complete customer problem?”
This approach reveals opportunities for 10x solutions that transform experiences entirely. As we’ve seen with touchscreens, fast desktop search, and app stores, markets tend to converge on optimal solutions, and companies that implement these solutions early can capture extraordinary value.
What if you could apply this same foresight to your market?
Consider the competitive advantage of anticipating where your industry will be in five years, while others make incremental improvements or chase technology trends (“We need AI!”) without clearly understanding the problems they solve. Imagine building a product roadmap based on validated insights rather than guesswork or following the hype cycle. The Deductive Innovation approach has potential to significantly impact an organisation’s strategic position and potential valuation.
The final article of this series provides a step-by-step framework to apply the key principles of market prediction to your industry. It covers how to:
Identify the fundamental customer problems in your industry
Map their interconnections
Assess where current solutions fall short of optimality
Envision the optimal solution toward which the market will converge
Validate your vision with early adopters
Position your product at the destination before competitors even see the path
Don’t miss this opportunity to transform how you think about product strategy and market positioning. Continue to the final piece of the series:
The 5-Step Framework to Predict and Create Your B2B SaaS Market’s Future
Learn how to systematically identify optimal solutions to customer problems and position your B2B SaaS at your market’s inevitable destination years ahead of competitors.
This is the final article in a 4-part series on predicting market evolution in B2B SaaS.
This was the third part of a 4-part series on market evolution and how to predict it using Deductive Innovation. Thanks for reading! Feel free to share the article with others:
Peter Thiel with Blake Masters: “Zero to One: Notes on Startups, or How to Build the Future”, Crown Business, 2014.
Ben Shneiderman: “Direct Manipulation: A Step Beyond Programming Languages”, Computer, 16(8), 57–69, 31 August 1983. https://www.cs.umd.edu/users/ben/papers/Shneiderman1983Direct.pdf
Priya Ganapati: "Verizon Signals the End of the Unlimited Data Plan", Wired, 21 June 2010, https://www.wired.com/2010/06/verizon-signals-the-end-of-the-unlimited-data-plan/
YouTube channel AppleVideoArchive’s video “Apple WWDC 2004”, https://www.youtube.com/watch?v=x51L6hbIxr4
A 2023 report estimated that App Store was 35% of Apple’s Services revenue in 2022, and in 2024 the Services revenue hit $100 billion, growing at double digits.
Gene Munster: “Apple’s App Store Is an Important Part of Its Profitability — And It’s Not Changing”, Deepwater Asset Management, August 11, 2023, https://deepwatermgmt.com/apples-app-store-is-an-important-part-of-its-profitability-and-its-not-changing/
Kif Leswing: “Apple’s services unit is now a $100 billion a year juggernaut after ‘phenomenal’ growth”, CNBC, October 31, 2024, https://www.cnbc.com/2024/10/31/apple-services-is-100-billion-per-year-juggernaut-but-growth-slowing.html
In 2007, Nokia’s net sales from mobile phones was about €25 billion, growing only at 1%, and operating margin was about 22%. Nokia’s Annual Report 2007, https://www.nokia.com/system/files/files/request-nokia-in-2007-pdf.pdf
All science fantasies can become reality one day, unless they go against fundamental laws of nature. It is only a question of our willingness, imagination, and persistence to discover the necessary knowledge and technology.
As David Deutsch puts it in Chapter 4 of The Beginning of Infinity (2011): “Before a discovery is made, no predictive process could reveal the content or the consequences of that discovery. For if it could, it would be that discovery. So scientific discovery is profoundly unpredictable.”
I don’t have first-hand experience of these products. In their marketing, almost all companies say they provide “unified, integrated solutions” but often the solutions are far from that ideal. Even less than optimal solutions can dominate markets until a superior solution emerges.
Luke Sophinos, tweet storm “Industry-specific vertical software companies will eat some of the most iconic internet businesses of the last decade”, August 7, 2023, https://x.com/lukesophinos/status/1688546801396416512