The 5-Step Framework to Predict and Create Your B2B SaaS Market’s Future (Part 4)
Learn how to systematically identify optimal solutions to customer problems and position your B2B SaaS at your market’s inevitable destination years ahead of competitors.
This is the final article in a four-part series on predicting market evolution in B2B SaaS. Read Part 1, Part 2, and Part 3 if you haven’t already.
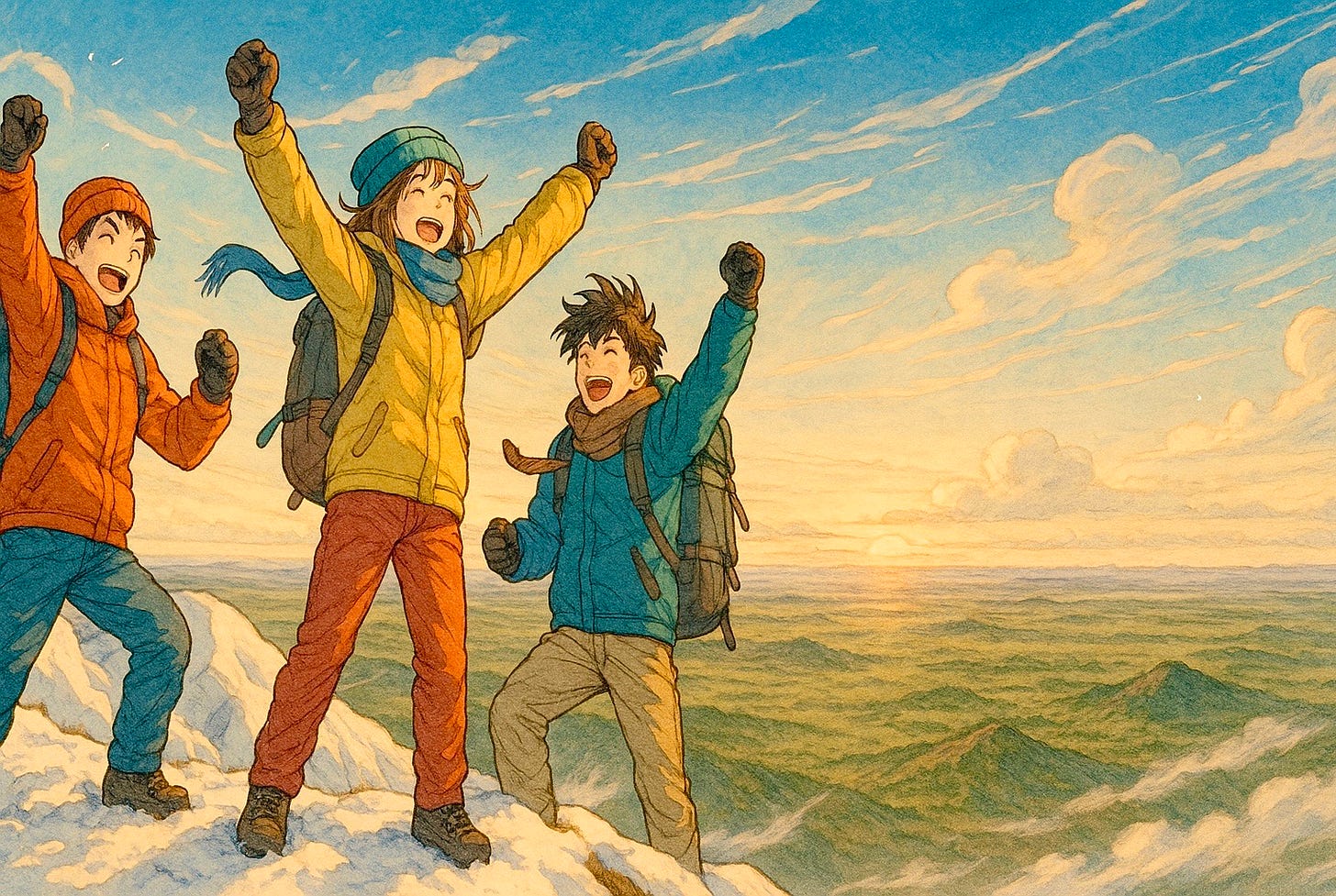
Throughout this series, we have established a powerful premise: markets tend to converge toward optimal solutions. Those who identify these solutions first gain extraordinary competitive advantages. But knowing this pattern exists isn’t enough. You need a systematic method to apply it to your specific B2B SaaS market.
This final article provides a practical 5-step framework to turn predicting your market to leading your market. You will learn how to:
Systematically identify fundamental customer problems in your target market
Map critical interconnections between these problems
Identify the largest gaps in current solutions
Envision the optimal solution that will eventually dominate your market
Validate your vision before investing significant resources
Unlike traditional approaches that leave product teams climbing whatever hill they happen to find, this framework enables you to identify your Mount Kilimanjaro – the highest peak in your market landscape – and plot your path there directly. Companies that master this skill don’t have to just respond to market evolution, they can create it.
For readers just joining this series: The previous articles established how markets converge toward optimal solutions, discussed why today’s best product development practices fail at market prediction, introduced Deductive Innovation as a systematic alternative, and showcased real-life examples of predicting major market shifts years ahead of time.
Now let’s examine how to apply these principles to your own market.
Five Strategic Steps to Create Your Market’s Future
How can you apply this prediction methodology to your own market and create its future? Here are five steps to get started applying basic principles of Deductive Innovation to market prediction.
1. Identify the fundamental customer problems in your industry
Begin by researching the stable, enduring problems that businesses in your target industry face. What are they trying to accomplish? What situations do they repeatedly encounter? What outcomes are they seeking?
Look deeper: What are their criteria for great outcomes? What aspects of their circumstances remain the same, with or without your solution?
The key is to discover, understand and document these problems independently of any software solutions. Don’t think about features, technologies, or existing products. Focus solely on the concrete situations, goals, and success criteria that exist regardless of how they are currently being addressed.
Discovering customer problems requires you to meet customers, talk to users, and observe how they solve their problems today. Particularly in B2B, the necessary information is not available on the internet nor can you interrogate ChatGPT to find it out. Field research is necessary.
Example 1: If you are building software for the construction industry, don’t start by asking what features they need. Instead, observe how construction projects are planned, how work progresses at construction sites, how project managers coordinate resources across sites, how they track progress against schedules and budgets, how they manage compliance documentation, how they coordinate with subcontractors, and so on. These fundamental activities have remained largely unchanged for decades, though the tools used have evolved.
Pay special attention to decision-making points. What information is needed to make optimal decisions, regardless of how well customers use that information today? What kind of knowledge, experience or judgement do humans apply in these decisions? You must understand all inputs and criteria of a decision-making process to actually improve it.
For the purpose of evaluating an opportunity, you need to observe what’s wrong with the existing tools and processes. You will need this information for positioning, marketing and selling.
However, to identify the customer problems, you should not pay much attention to all the details of how current tools and processes work, even though this is easy to observe. Why? Because a new solution can overhaul existing tools and processes completely.
Instead keep asking: what about the work and situations here cannot change regardless of what the solutions are like?
Example 2: When we applied this to the telco configuration case from Part 3, we discovered the situations behind the request for “faster import/export”: engineers needed to make a large number of interdependent changes to service configurations, test them, and change them. This customer problem persisted regardless of what tools they used.
Document specific examples of real situations, not just abstract descriptions. Take a lot of photos and/or videos, record the interviews, and transcribe the recordings. Collect data samples. Ask questions when you don’t understand why people are doing what they are doing. The concrete details reveal opportunities that general statements miss, and are required for objective evaluation and solution deduction.
2. Map the interconnections between these problems
Business problems are interconnected. Each process output becomes another’s input. Mapping these connections helps identify natural boundaries for integrated solutions.
Example 1: In manufacturing, production planning influences material procurement, which affects inventory management, which impacts shipping logistics.
Example 2: In the construction industry, scheduling, resource allocation, and material ordering form a tightly interconnected problem set. When a construction manager changes the schedule, it affects which workers and equipment are needed on which days, which then impacts material delivery timing. A typical current solution forces managers to manually coordinate these interconnections across separate systems.
Understanding these links reveals optimal solution scope. The stronger the interdependencies, the more customers can benefit from a holistic, integrated solution.
Customer problems that have fast feedback loops between them should always be solved together.
Example 3: Consider a university student planning her semester. She must coordinate course selection (problem 1) with her weekly schedule (problem 2). Prerequisites and degree requirements determine which courses she could take. Course choices affect her schedule through lecture times, exercise sessions, and exams. But her schedule limits which courses she can actually take due to time conflicts. An optimal solution lets her plan both the course selection and the schedule simultaneously and see how decisions affect her overall plan.
Interconnected customer problems with slower feedback loops between them should also be researched upfront, even though they don’t necessarily need to be solved upfront. It’s sufficient to just identify ahead of time the necessary inputs and outputs to support optimal outcomes for each interconnected problem. That enables us to ensure that the separate solutions for the separate subproblems will work together optimally once completed later.
An example of a typical slower feedback loop is the learning cycle. Most businesses have two parallel sets of business processes: processes to plan the operations, and the actual business operations. To make better plans in the future, a planning process needs historical data from operations to analyse how well previous plans worked and to estimate the impact of new plans, relative to goals. Establishing the collection and interchange of all the right data as an afterthought is likely to pose major architectural challenges.
Example 4: Production planning of manufacturing operations must anticipate future demand and estimate how long the manufacturing process will take. Patterns of past orders are necessary input for forecasting future orders, and actual results from past manufacturing processes are necessary to estimate the throughput and lead time of the manufacturing process going forward.
To map interconnections effectively:
Identify necessary dependencies between different activities, regardless of current tools and processes
Note where outputs from one process become inputs to another, or should become inputs to another to enable optimal outcomes
Map all the decision points where changes in one area will impact others
Pay special attention to processes that require frequent coordination
Document the costs (time, effort, errors) of poor integration for opportunity evaluation
The strongest interconnections reveal opportunities for integrated solutions that will inevitably emerge in the market.
3. Assess the current solution landscape
Now look at the solutions that customers are using in your market. What products, software and ad hoc tools are they using today? How well do the existing solutions address the concrete situations you have identified? How good decisions and outcomes do they enable? How cumbersome is the process to reach the outcomes? Where are the gaps? Where is unnecessary complexity, usually imposed by bad tools and processes? Where are integrations and hand-offs particularly painful?
Deductive Evaluation provides methods to objectively evaluate existing solutions and measure improvement potential. However, the ability to separate problems from solutions and common sense will get you quite far here.
This assessment helps you identify the biggest opportunities for improvement. The areas with the largest gap between current solutions and optimal solutions are where market disruption is most likely to occur.
Example 1: Software installation in the early 2000’s highlighted this gap well. Secure solutions required users to understand digital certificates and make complex security decisions that no ordinary user could do. The easiest approaches (downloading EXE files and clicking through defaults) were potential security disasters. Users clicked through installation wizards, accepting whatever defaults appeared. The gap between “secure” and “usable” was gaping wide open, and our analysis indicated that a centralised distribution model could close the gap. That solution ultimately materialised in app stores.
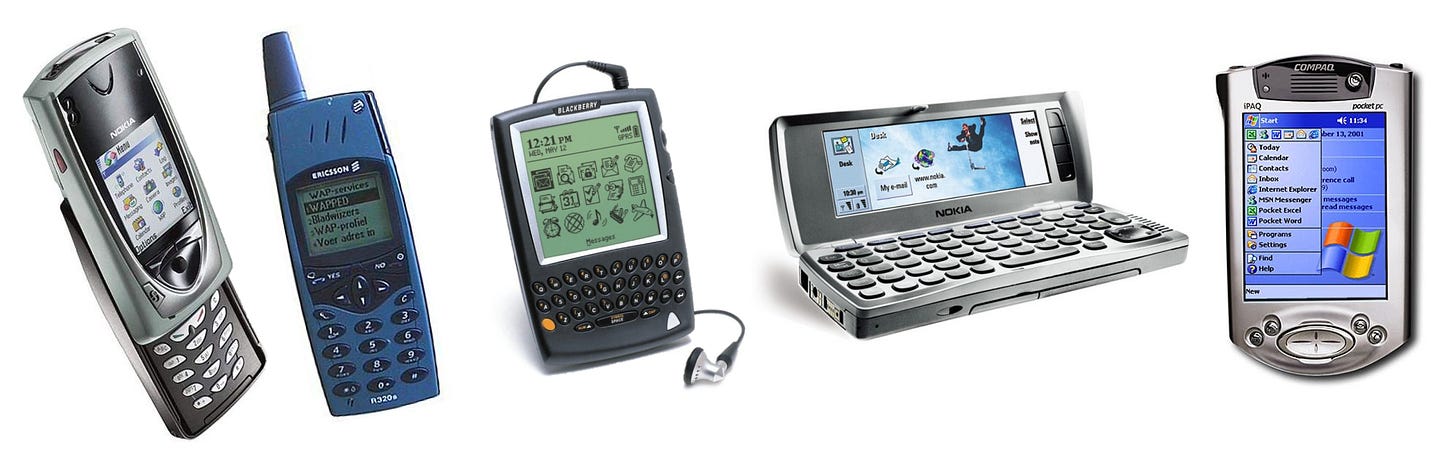
Example 2: When we analysed mobile computing also in the early 2000’s, we saw a landscape of disconnected solutions: PDAs with styluses without internet connection, WAP phones with tiny screens and number pads, and smart phones with reasonably sized screens, but without touchscreens. None of them made it easy to access and interact with internet content in a direct and easy way when users were away from a desk.
Be particularly attentive to:
Point solutions that address only one part of an interconnected problem set
Processes that require many manual steps between different systems
Quality of the outcomes customers are able to reach
Areas where customers have built elaborate workarounds
Functionality that requires extensive configuration to be useful
Features that exist but are rarely used
High-value workflows that span multiple current solutions
This analysis will reveal the most significant opportunities for optimisation in your market.
4. Envision the optimal solution
Based on your understanding of the problem space, what would an optimal solution look like? Not just better than current options, but a truly optimal one that solves the complete customer problem with minimal complexity?
Be bold here. Don’t constrain your thinking to incremental improvements of existing solutions. Imagine designing a solution from scratch with today’s cutting edge technology, unconstrained by today’s approaches.
Ask questions like:
If we were designing this system today with no legacy constraints, what would it look like?
What do the ideal outcomes look like at each point of the overall process?
What would be the ideal workflow that contains only activities that are necessary to reach ideal outcomes?
What information or functionality should be available at each decision point to enable optimal decisions?
What are the activities that humans must necessarily complete because they have knowledge, understanding, or judgement that a computer lacks, even if programmed with today’s best AI technology? (The rest can be automated.)
How could we unify what are currently separate tools or systems?
This vision becomes your North Star, the peak of your Kilimanjaro, the global maximum, the destination where your market will eventually arrive.
The more thoroughly you’ve understood the fundamental customer problems, the more accurate your vision can be.
Example: For mobile app installation, the optimal solution had to solve both security and usability together. Security was even more important on mobile, because phones could be exploited to make expensive calls and we expected mobile payments would soon become common. Rather than improving warnings or technical certificates, we envisioned a completely different approach: a central distribution model with financial and legal accountability mechanisms that eliminated security decisions for users entirely.
5. Validate your vision with early adopters
Finally, test your vision with potential early adopters. Like I described validation in Part 3, this doesn’t require building the solution. At the first stage, validate problems across customers, and at the second stage, validate your solution concept.
When you are validating customer problems (=customers’ situations) describe your understanding of their situation in detail. Look for a reaction where the customer’s eyes light up as they recognise themself and their situation, and say “That’s right!” without adding anything to your description. If they keep correcting you or adding to what you said you haven’t yet understood the full picture.
To validate the solution, create scenarios, mockups, or prototypes that illustrate how your envisioned solution would work in specific customer situations – the same ones you have first discovered.
Example: Recall the founder from Part 3 who presented a solution concept to a prospect. Without building a product and just by demonstrating how their solution would address the customer’s validated problems, the customer said, in effect, “This is so good that you have to build it” and offered to finance product development.
When you get a similar reaction, you are on the right track. Get the customer to sign a commitment, at least a conditional one, to pay for the solution. That’s how you know you’ve identified an opportunity worth pursuing relentlessly.
Effective validation focuses on:
Whether your problem descriptions match their reality
If your solution concept addresses their highest-value problems
How much better your approach is than current alternatives
Their willingness to pay for and champion such a solution
Any critical gaps or misunderstandings in your concept
The goal isn’t 100% perfect prediction, but rather identifying where the market is heading with enough accuracy to position your product at the forefront of that evolution.
Why Most Product Leaders Doubt Market Prediction (And Why They’re Wrong)
After presenting this market prediction approach across four articles, you might still have concerns. Let me address common objections that I usually hear from product leaders and other software professionals.
“Markets are fundamentally unpredictable”
While major technological breakthroughs are generally unpredictable (as we saw with LLMs), how existing technologies get applied to solve customer problems follows consistent patterns. Markets converge toward solutions that address complete problems with minimal complexity. This pattern is what makes prediction possible.
“This sounds too theoretical without real-world testing”
The examples in Part 3 (from touchscreens to app stores) demonstrated that systematic analysis of customer problems consistently predicted market evolution years in advance. The validation steps in this framework ensure your predictions connect to market reality before you make significant investment.
“What about disruptive technologies?”
As acknowledged in Part 3, this approach predicts how existing technologies will be applied, not what radically new technologies will emerge. However, understanding fundamental customer problems positions you to rapidly adapt new technologies when they appear, as the problems themselves remain stable.
“Our industry is too complex for this approach”
Complex industries offer the greatest opportunities for 10x improvements. When current solutions are convoluted precisely because the domain is complex, systematic analysis is most likely to reveal drastically better, more direct paths to customer value.
“We don’t have time for this analysis”
As covered in Part 2, spending a few weeks or months on discovery with a small team of 1-3 people can prevent years of building the wrong product.
However, I need to be candid: this approach is not for everyone. If the company culture values quick action over strategic thinking, emphasises incremental improvements over revolutionary advancements, and supports a development horizon of just a quarter instead of years, this approach won’t fit. Deductive Innovation requires a long-term mindset and genuine ambition for excellence. For those committed to building category-defining products and willing to invest upfront thinking to achieve 10x results, the ROI can be dramatic.
For everyone else, traditional agile iteration is more culturally compatible, even if less effective.
“What if the technology isn’t ready for the optimal solution?”
Sometimes the optimal solution requires technology that isn't mature enough. In 2001, capacitive multitouch technology wasn’t ready to enable an optimal touchscreen interface. However, understanding what the optimal solution requires helps companies recognise when enabling technologies emerge. Apple acquired FingerWorks in early 2005 precisely because they understood what the optimal mobile interface needed.1 Companies that clearly see the destination can either develop missing technologies or recognise and adopt them when available.
“Our legacy architecture prevents implementing optimal solutions”
For established companies, this might be the most challenging reality. When existing product architecture has evolved from years of suboptimal decisions and portfolio acquisitions, implementing even small optimal improvements tend to require relatively big architectural changes. To quote an old Finnish idiom, it’s like a magpie stuck on a tarred roof: when the tail lifts, the beak sticks. Every attempt to make some part optimal ripples through the complex interconnected system.
This is why starting toward optimality from the beginning matters so much.
Companies with complex legacy products face a strategic choice:
continue making incremental improvements within architectural constraints, or
invest in revamping the architecture to support the eventual optimal solution.
In the short run, the former seems like a rational choice. This is the famous innovator’s dilemma.2 But doing everything right according to traditional business thinking (making incremental improvements, serving the best existing customers, maintaining profit margins) can lead to failure when a competitor without legacy constraints builds the optimal solution, and eventually dominates the market.
Sometimes the wisest strategic decision is to disrupt yourself before someone else does.
Choosing the Right Mountain: Strategic Application of Market Prediction
Many companies that will dominate B2B and Enterprise SaaS markets in the coming years won’t be those with the most developers, biggest marketing budgets, or even the most advanced technology. They will be those that can systematically find optimal solutions through deep understanding of customer problems in their industry. Once on the right mountain, they plot the most efficient path to the top and climb relentlessly.
Deductive Innovation answers the strategic question: “Which mountain should we climb?”
Traditional iterative approaches help execute: “How should we climb it efficiently?”
Both are necessary, but the sequence matters critically. Iterating before you have identified the right mountain can lead to years of wasted effort and missed opportunities.
This strategic advantage of Deductive Innovation comes from three capabilities most of your competitors lack:
Measurement before building: You can measure alternative solutions analytically before building anything, eliminating expensive development of solutions that create insufficient customer value
Direct definition without endless iteration: You can define optimal solutions directly from validated customer problems, cutting months or even years from your development timeline and positioning your product where the market will inevitably converge.
10x superior solutions customers immediately recognise: You can create solutions with 10x better Problem-Solution Fit that customers immediately recognise as superior, dramatically reducing sales cycles and customer acquisition costs while enabling premium pricing.
As you consider your own product strategy, ask yourself:
Are you seeing the entire landscape of customer problems in your industry?
What would the optimal solution look like if you started fresh?
How would you proceed to building one well-designed, thoroughly thought-through vertical SaaS platform that solves your customers’ problems holistically?
How close is your current roadmap to building that optimal solution?
Most importantly: Are you climbing the right mountain?
While this article series provides an approach for market prediction, implementing Deductive Innovation effectively requires more methodological knowledge than I can cover in a single series. Adopting this systematic zero-to-one methodology challenges many ingrained beliefs in technology companies, where the tendency is to jump quickly to solutions and technology. Effective adoption involves:
Developing specialised problem discovery techniques
Learning analytical evaluation methods
Mastering the process of deducing optimal solutions
If you are interested in learning more about these methodologies, subscribe to this newsletter where I will continue to share insights on systematic B2B software innovation.
Leading Your Market's Evolution: The Power of Systematic Prediction
Throughout this series, we have seen how markets follow predictable patterns of evolution. Companies that understand this pattern don’t just survive, they define where entire industries go next.
We have established that:
Markets converge toward optimal solutions that address complete customer problems with minimal complexity
Traditional approaches fail because they iterate without knowing the destination
Systematic analysis reveals that destination before competitors see it
This approach has successfully predicted major market shifts years in advance, from touchscreens smartphones to app stores
The framework presented in this article gives you practical steps to apply these insights to your own market:
Discover the fundamental problems your customers face
Map their interdependencies
Evaluate where current solutions fall short
Envision the optimal solution to the complete customer problem
Validate with early adopters before significant investment
Execute with confidence
The insight at the heart of this approach is profound: by understanding customer problems deeply and reasoning through their optimal solutions, you can see where your market is heading.
You don’t need luck or genius, just a systematic framework for uncovering what others miss. Remember that the map to your Kilimanjaro is not found in customer feedback, competitive analysis, or industry trends. It’s encoded in the stable customer problems that have persisted for years and even decades. While your competitors remain focused on feature requests and iterating towards a hilltop, you can identify the global maximum they miss, and position your product where the rest of the market will eventually arrive.
Will you use this framework to shape your market’s future rather than chase it?
If you’re ready to apply these principles to your B2B SaaS product, subscribe now for more insights on creating superior B2B SaaS products or get in touch for a consultation.
This concludes our four-part series on predicting market evolution in B2B SaaS. If you found these insights valuable, share the article with your colleagues!
Juliana Reyes: “How I sold my company to Apple: Jeff White, former FingerWorks CEO [Q&A]”, Technically Media, Jan 9, 2013, https://technical.ly/startups/jeff-white-fingerworks-apple-touchscreen/
Clayton M. Christensen: “The Innovator’s Dilemma: When New Technologies Cause Great Firms to Fail”, Boston, MA: Harvard Business School Press, 1997, https://www.hbs.edu/faculty/Pages/item.aspx?num=46